Growth/Personalized first day/構造化タスク/リンクの追加
このページでは Growth チームが手がける構造化タスク「リンクの追加」について説明します。これはGrowth チームが新規参加者ホームページで提供する構造化タスクのひとつです。 このページでは主要なアセット、設計、未決の課題、意思決定について述べます。 進行中の増分更新のほとんどは全般的なGrowthチームの更新ページに投稿されます。このページにはいくつかの大規模または詳細な更新を掲載します。
Add a link
![]() Suggest potential wikilinks as easy edits for newcomers
|
2021年8月時点で、このタスクの最初のイテレーションがアラビア語版、チェコ語版、ベトナム語版、ブラジル語版、ベンガル語版、ポーランド語版、フランス語版、ロシア語版、ルーマニア語版、ハンガリー語版、およびペルシャ語版ウィキペディアの全新規登録アカウントの半数に実装されました。 機能の実装から 2週間に集計したデータを解析したところ、新規参加者はこれらの編集をたくさんこなしており、そしてその差し戻し率は低いとわかりました。 この分析からわかった事柄は機能の改善に活用され、成果が現れてもっと多くのウィキに実装するまでになりました。
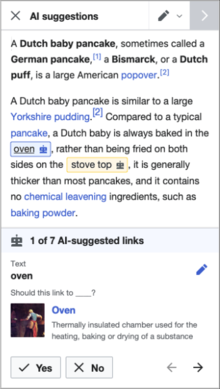
我々が構築している対話式の試作品をご覧ください。 試作版なので、すべてのボタンが動作するわけではないことに注意してください:
チームのメンバーがウィキマニア2021において、この取り組みの背景、アルゴリズム、実装および成果を発表しました。 動画はこちら。 and the スライド集はこちら。
現状
- 2020-01-07: リンク推奨アルゴリズムの実行可能性の最初の評価
- 2020-02-24: 改良したリンク推奨アルゴリズムを評価
- 2020-05-11: 構造化タスクとリンク推奨についてコミュニティと議論
- 2020-05-29: 最初のワイヤーフレーム
- 2020-08-27: バックエンドの開発作業に着手
- 2020-09-07: モバイル設計の初回の利用者テスト
- 2020-09-08: 設計最新版について、コミュニティに協議を呼びかける
- 2020-10-19: モバイル設計の第2回の利用者テスト
- 2020-10-21: デスクトップ設計の初回の利用者テスト
- 2020-10-29: フロントエンドの開発作業に着手
- 2020-11-02: デスクトップ設計の第2回の利用者テスト
- 2020-11-10: アラビア語、ベトナム語、チェコ語のコミュニティから設計へのフィードバックを呼びかけ
- 2021-04-19: 用語集と数値についての節を追加
- 2021-05-10: 先行ウィキ4件で機能をテスト
- 2021-05-27: アラビア語版、ベトナム語版、チェコ語版、およびベンガル語版ウィキペディアにおいて、新規参加者の半数に実装
- 2021-07-21: ポーランド語版、ロシア語版、フランス語版、ルーマニア語版、ハンガリー語版、およびペルシャ語版ウィキペディアにおいて、新規参加者の半数に実装。
- 2021-07-23: 機能実装から最初の2週間における分析を公表。
- 2021-08-15: 背景、実装、アルゴリズム、および成果に関してウィキマニアで発表。
- 2022-05-20: 機能のイテレーション2の完了、これにはコミュニティのフィードバックとデータ分析に基づく改善が含まれる。(リンクの追加「イテレーション2」ページの改善の一覧を参照)。
- 2022-06-19: 「リンクの追加」実験分析を公開
- 2022-08-20: 構造化タスクに関する巡回者のフィードバックに対処する作業を開始 (T315732)
- 2022-09-02: 新規参加者タスクの編集の種類の分析を公開
- 2023-12-31: Almost all Wikipedias now have this task available for newcomers. The exception are a dozen of small wikis where there are not enough articles to activate the algorithm, and English and German Wikipedias.
- 次: すべてのウィキに展開し、巡回者と協力してリンクの編集に取り組む体験を改善する。
要約
構造化タスクは編集タスクを段階ごとのワークフローに分割して、新規参加者に理解しやすく、そしてモバイル機器で理解しやすいようにすることを目的としています。 Growthチームは、これら新しい種類の編集ワークフローによって、より多くの新規参加者がウィキペディアへの関与を始めやすくなり、その中にはより実質的な編集を学んでコミュニティへ参加する人も出てくるだろうと信じています。 構造化タスクのアイデアをコミュニティと議論したのち、チームでは最初の構造化タスクの構築を決めました:それが「リンクの追加」です。 このタスクはウィキリンクすると良いかもしれない単語や成句を指摘するアルゴリズムを使用する予定であり、新規参加者は提案を受け入れるか却下することができます。 このプロジェクトで、これらの疑問について学びを得たいと考えています:
- 構造化タスクは新規参加者にとって魅力的ですか?
- 新規参加者はモバイルで構造化タスクがうまくいきますか?
- 価値のある編集を生み出しますか?
- 新規参加者の関与を増やすことにつながりますか?
「リンクの追加」実験分析の完了後、「リンクの追加」構造化タスクは、特に建設的な(差し戻されない)編集に関しては、Growth機能を使用できない対照群だけでなく、構造化されていない「リンクの追加」タスクを使用できる群よりも新規参加者の成果が改善すると結論付けることができました。特に重要な点は:
- 「リンクの追加」構造化タスクを提供された新規参加者は、より活性化する傾向にあります(つまり建設的な最初の記事編集をします)。
- またより定着する傾向にあります(つまり別の日に戻ってきて他の建設的な記事編集をします)。
- またこの機能は、編集量(つまり最初の2週間にわたり行った建設的な編集の回数)を増やし、同時に編集の品質(つまり新規参加者の編集が差し戻される傾向)を改善します。
なぜウィキリンクか?
以下は構造化タスクページからの引用で、なぜ「リンクの追加」を最初の構造化タスクとして選ぶのか説明しています。
Growthチームは現在のところ(2020年5月)「リンクの追加」ワークフローを上表で一覧にした他のものよりも優先したいと考えています。 「校正」のような、他のワークフローがより価値がありそうではありますが、まず「リンクの追加」から始めたい理由がいくつかあります:
- 短期的には、まずやりたい重要なことは「構造化タスク」がうまくいくというコンセプトを証明することです。 したがって、最初のバージョンにあまり多くの投資をする必要なしに、利用者に展開して再び学ぶことができるように、最も単純なものを構築したいです。 最初のバージョンがうまくいけば、より構築するのが難しい様々なタスクに投資する自信が持てるでしょう。
- 「リンクの追加」は我々にとって構築するのが最も簡単そうです。なぜならば、WMF 研究チームによって構築されたアルゴリズムが既にあって、ウィキリンクの提案をうまくやっているようだからです(アルゴリズムの節をご参照ください)。
- ウィキリンクの追加で新規参加者はたいてい自分で何もタイプする必要がなく、それによって我々が設計および構築すること -- そして新規参加者が完遂することが非常に簡単になるだろうと考えています。
- ウィキリンクの追加はリスクが低そうな編集です。 言い換えれば、間違ったリンクの追加によって記事の内容が損なわれる可能性は、間違った出典や画像の追加によって記事の内容が損なわれる可能性ほどはありません。
設計
この節には現在検討中の設計が含まれます。 「リンクの追加」構造化タスクの設計に関するすべての考えを見るには、背景、ユーザーストーリー、および初期設計コンセプトを含む、このスライドショーをご覧ください。
複数回の利用者テストとイテレーションを通して、我々の設計は進化しました。 2020年12月現在、この機能の最初のバージョンとして開発する予定の設計は決まっています。 これらの対話式の試作品をご覧ください。 試作版なので、すべてのボタンが動作するわけではないことに注意してください:
比較レビュー
機能を設計するときに、ウィキメディア以外の世界にある他のソフトウェアプラットフォームで類似の機能を調べます。 これらはAndroidの提案された編集機能で行われた比較レビューから浮き彫りになった点の一部で、我々のプロジェクトに関連性があるものです。
- Task types – are divided into five main types: Creating, Rating, Translating, Verifying content created by others (human or machine), and Fixing content created by others.
- Visual design & layout – incentivizing features (stats, leaderboards, etc) and onboarding is often very visually rich, compared to pared back, simple forms to complete short edits. Gratifying animations often compensate for lack of actual reward.
- Incentives – Most products offered intangible incentives grouped into: Awards and ranking (badges) for achieving set milestones, Personal pride and gratification (stats), or Unlocking features (access rights)
- Users motivations – those with more altruistic motivations (e.g., help others learn) are more likely to be incentivized by intangible incentives than those with self-interested motivations (e.g., career/financial benefits)
- Personalization/Customization – was used in some way on most apps reviewed. The most common customization was via surveys during account creation or before a task; and geolocalization used for system-based personalization.
- Guidance – Almost all products reviewed had at least basic guidance prior to task completion, most commonly introductory ‘tours’. In-context help was also provided in the form of instructional copy, tooltips, step-by-step flows, as well as offering feedback mechanisms (ask questions, submit feedback)
Initial wireframes
After organizing our thoughts and doing background research, the first visuals in the design process are "wireframes". These are simply meant to experiment and display some of the ideas we think could work well in a structured task workflow. For full context around these wireframes, see the design brief slideshow.
-
Giving more explanation the first time the newcomer uses the workflow than on subsequent times
-
Explaining why the task is worth doing
-
Teaching concepts through checklists
-
Letting users disagree with algorithms and generate useful data in the process
-
Encouraging the newcomer to move on to more impactful tasks and learn more
-
Providing opportunities to learn the more powerful editing tools
-
Guardrails against low-quality contributions
-
Rewarding good work
モバイルの模型:2020年8月
我々のチームは前節からのワイヤーフレームについて議論しました。 コミュニティのメンバーによって述べられた好みを考慮に入れ、技術的制約について考えると、新規参加者にとって何が最善だろうかと検討しました。 2020年8月、機能がどのようになるのかより詳細に示すことを目指して、模型の作成を次の段階に進めました。 これらの模型(あるいは類似のバージョン)はチームの議論、コミュニティの議論、および利用者テストで使われる予定です。 これらの模型について我々が考えている最も重要なことのひとつは、議論の間コミュニティのメンバーから一貫して聞いた懸念です:構造化タスクは新規参加者に編集を紹介する良い方法かもしれませんが、新規参加者が従来の編集インターフェースにも興味があれば、確実に見つけて使用できるようにしたいです。
2つの異なる設計コンセプトについて模型があります。 必ずしも、どちらかひとつの設計コンセプトを選ぶことを目的としていません。 どちらかといえば、2つのコンセプトは異なるアプローチを実演することを意図しています。 設計の最終案は、両方のコンセプトから良いところを取り入れるかもしれません。
- コンセプトA:ビジュアルエディターで構造化タスク編集を実施します。 「おすすめモード」からソースエディターまたはビジュアルエディターモードに切り替えて、利用者が記事全体を見ることができます。 リンクの追加にあまり焦点を当てていませんが、ビジュアルエディターとソースエディターにより簡単にアクセスできます。
- コンセプトB:新しい独自の場所で構造化タスク編集を実施します。 記事の中で注目する必要がある段落のみを利用者に表示し、選択すれば記事の編集に進むことができます。 リンクの追加から気をそらすものはより少ないですが、ビジュアルエディターやソースエディターによりアクセスしづらいです。
この2つの模型は、単語や言語ではなく、利用者の流れや体験に焦点を当てていることに注意してください。 今後、機能に書く文はどうするか、また利用者にリンクを追加するべきかどうか説明するため、最善の方法を検討します。
Static mockups
これらの設計コンセプトを見るには、以下のスライドの全セットを見ることをお勧めします。
Interactive prototypes
You can also try out the "interactive prototypes" that we're using for live user tests. These prototypes, for Concept A and for Concept B, show what it might feel like to use "add a link" on mobile. They work on desktop browsers and Android devices, but not iPhones. Note that not everything is clickable -- only the parts of the design that are important for the workflow.
根源的な質問
これらの設計を議論する時、私たちのチームでは、いくつかの根源的な質問に回答を得たいと望んでいます。
- 編集は記事で行うべきですか(文脈がより多い)?それとも、この種類の編集のために専用の体験を設計すべきですか(より焦点を当てられるが、エディタの使用との隔たりが大きい)?
- もしもリンク先またはリンクのテキストを編集をしたい人が現れたらどうなるでしょうか?阻止すべきでしょうか、あるいは通常のエディタへ誘導すべきでしょうか?これはビジュアルエディタについて教える好機でしょうか?
- 新規参加者に従来の編集ツールを見つけてもらうには、サポートが肝心であることを承知しています。 しかし、そのタイミングは?構造化タスクを体験している間に、エディタに移行できることをお知らせしますか?それとも、特定の数の構造化タスクをこなした後のように、マイルストーンを達成したときに定期的に?
- ここで「ボット」という単語は適切でしょうか? 言い換えは可能でしょうか? ひょっとすると「アルゴリズム」「コンピュータ」「機械」「自動◯◯」などは使えそう? 機械がお勧めするものには間違いがあり、人間が考える重要性を伝えるには 、表現を改善できませんか?
Mobile user testing: September 2020
Background
During the week of September 7, 2020, we used usertesting.com to conduct 10 tests of the mobile interactive prototypes, 5 tests each of Concepts A and B, all in English. By comparing how users interact with the two different approaches at this early stage, we wanted to better understand whether one or the other is better at providing users with good understanding and ability to successfully complete structured tasks, and to set them up for other kinds of editing afterward. Specific questions we wanted to answer were:
- Do users understand how they are improving an article by adding wikilinks?
- Do users seem like they will want to cruise through a feed of link edits?
- Do users understand that they're being given algorithmic suggestions?
- Do users make better considerations on machine-suggested links when they have the full context of the article (like in Concept A)?
- Do users complete tasks more confidently and quickly in a focused UI (like in Concept B)?
- Do users feel like they can progress to other, non-structured tasks?
Key findings
- The users generally were able to exhibit good judgment for adding links. They understood that AI is fallible and that they have to think critically about the suggestions.
- While general understanding of what the task would be ("adding links") was low at first, they understood it well once they actually started doing the task. Understanding in Concept B was marginally lower.
- Concept B was not better at providing focus. The isolation of excerpts in many cases was mistaken for the whole article. There were also many misunderstandings in Concept B about whether the user would be seeing more suggestions for the same term, for the same article, or for different articles.
- Concept A better conveyed expectations on task length than Concept B. But the additional context of a whole article did not appear to be the primary factor of why.
- As participants proceed through several tasks, they become more focused on the specific link text and destination, and less on the article context. This seemed like it could lead to users making weak decisions, and this is a design challenge. This was true for both Concepts A and B.
- Almost every user intuitively knew they could exit from the suggestions and edit the article themselves by tapping the edit pencil.
- All users liked the option to view their edits once they finished, either to verify or admire them.
- “AI” was well understood as a concept and term. People knew the link suggestions came from AI, and generally preferred that term over other suggestions. This does not mean that the term will translate well to other languages.
- Copy and onboarding needs to be succinct and accessible in multiple points. Reading our instructions is important, but users tended not to read closely. This is a design challenge.
Outcome
- We want to build Concept A for mobile, but absorbing some of the best parts of Concept B's design. These are the reasons why:
- User tests did not show advantages to Concept B.
- Concept A gives more exposure to rest of editing experience.
- Concept A will be more easily adapted to an “entry point in reading experience”: in addition to users being able to find tasks in a feed on their homepage, perhaps we could let them check to see if suggestions are available on articles as they read them.
- Concept A was generally preferred by community members who commented on the designs, with the reason being that it seemed like it would help users understand how editing works in a broader sense.
- We still need to design and test for desktop.
Ideas
The team had these ideas from watching the user tests:
- Should we consider a “sandbox” version of the feature that lets users do a dry run through an article for which we know the “right” and “wrong” answers, and can then teach them along the way?
- Where and when should we put the clear door toward other kinds of editing? Should we have an explicit moment at the end of the flow that actively invites them copyedit or do another level task?
- It’s hard to explain the rules of adding a link before they try the task, because they don't have context. How might we show them the task a little bit, before they read the rules?
- Perhaps we could onboard the users in stages? First they learn a few of the rules, then they do some links, then we teach them a few more pointers, then they do more links?
- Should users have a cooling-off period after doing lots of suggestions really fast, where we wait for patrollers to catch up, so we can see if the user has been reverted?
Desktop mockups: October 2020
After designing, testing, and deciding on Concept A for mobile users, we moved on to thinking about desktop users. We again have the same question around Concepts A and B. The links below open interactive prototypes of each, which we are using for user testing.
- Concept A: the structured task takes place at the article, in the editor, using some of the existing visual editor components. This gives users greater exposure to the editing context and may make it more likely that they explore other kinds of editing tasks.
- Concept B: the structured task takes place on the newcomer homepage, essentially embedding the compact mobile experience into the page. Because the user doesn't have to leave the page, this may encourage them to complete more edits. They could also see their impact statistics increase as they edit.
We are user testing these designs during the week of October 23. See below for mockups showing the main interaction in each concept.
-
Mockup of Concept A
-
Mockup of Concept B
Outcome
The results of the desktop user tests led us to decide on Concept A for desktop for many of the same reasons we chose Concept A for mobile. The convenience and speed of Concept B did not outweigh the opportunity for Concept A to expose newcomers to more of the editing experience.
Terminology
"Add a link" is a feature in which human users interact with an algorithm. As such, it is important that user have a strong understanding that suggestions come from an algorithm and that they should be regarded with skepticism. In other words, we want the users to understand that their role is to evaluate the algorithm's suggestion and not to trust it to much. Terminology (i.e. the words we use to describe the algorithm) play an important role in building that understanding.
At first, we planned to use the terms "artificial intelligence" and "AI" to refer to the algorithm, but we eventually decided to use the term "machine". This may be a practice that gets adopted more broadly as multiple teams build more structured tasks that are backed by algorithms. Below is how we thought about this decision.
Background
As we build experiences that incorporate augmentation, we are thinking about the terminology to use when referring to suggestions that come from automated systems. If possible, we want to make a smart choice at the outset, to minimize changes and confusion later. For instance, we are looking at sentences in the feature like these:
- "Suggested links are machine-generated, and can be incorrect."
- "Links are recommended by machine, and you will decide whether to add them to the article."
Objectives
We want the terms we use to satisfy these objectives.
- Transparency: users should understand where recommendations come from, and we should be being honest with them.
- Human-in-the-loop: users should understand that their contributions improve recommendations in the future.
- Usability: copy should help users complete the tasks, not confuse or burden them with too much information.
- Consistency: we should use the same copy as much as possible to lower cognitive load.
Terms we considered
Term | Strengths | Weaknesses |
AI |
|
|
Machine |
|
|
Computer |
|
|
Algorithm |
|
|
Automated |
|
|
Methods and findings
- User testing: the Growth team tested "add a link" in English using the terms "artificial intelligence" and "AI". We found that users understood the term well and that English-speaking users understood that they should regard the output of AI with skepticism.
- Experts: we spoke to WMF experts in the fields of artificial intelligence and machine learning. They explained that the link recommendation is not truly "AI", in the way that the term is used in the industry today. They explained that by using that term, we may be over-inflating our work and giving users a false sense of the intelligence of the algorithm. Experts preferred the term "machine", as it would accurately describe the link recommendation algorithm as well as be broad enough to describe almost any other kind of algorithm we might use for structured tasks.
- Multi-lingual community members: we spoke to about seven multilingual colleagues and ambassadors about the terms that would make the most sense in their languages. Not all languages have a short acronym for "AI"; many have long translations. The consensus was that "machine" made good sense in most languages and would be easy to translate.
Result
We are going to use the term "machine" to refer to the link recommendation algorithm, e.g. "Suggested links are machine-generated". See screenshots below to see one of the places where the terminology changed based on this decision.
-
Onboarding screen using the term "AI"
-
Onboarding screen using the term "machine"
Measurement
Hypotheses
The “add a link” workflow structures the process of adding wikilinks to a Wikipedia article, and assists the user with artificial intelligence to point out the clearest opportunities for adding links. Our hypothesis with the “add a link” workflow is that such a structured editing experience will lower the barrier to entry and thereby engage more newcomers, and more kinds of newcomers than an unstructured experience. We further hypothesize that newcomers with the workflow will complete more edits in their first session, and be more likely to return to complete more.
Below are the specific hypotheses we seek to validate. These govern the specifics around which data we'll collect and how we'll analyze it.
- The "add a link" structured task increases our core metrics of activation, retention, and productivity.
- “Add a link” edits are more likely to be successful than unstructured suggested edits, meaning that a user completes the task and saves the edit. They are also more likely to be constructive, meaning that the edit was not reverted, than unstructured suggested edits.
- Users seem to understand this task more than unstructured tasks.
- Users who start with "add a link" will move on to other kinds of tasks, instead of staying siloed (the latter being a primary community concern).
- The perceived quality of the link recommendation algorithm will be high, both from the users who make "add a link" edits and the communities who review those edits.
- Users who get “add a link” and who primarily use/edit wikis on mobile see a larger increase in the effects on retention and productivity relative to desktop users.
Experiment Plan
A randomly selected half of users who get the Growth features will get "add a link" tasks, and the other randomly selected half will get unstructured link tasks. We prefer to give users maximum exposure to these tasks and will therefore not give these users any copyedit tasks by default. In other words, for the purposes of this experiment, we’ll change the default difficulty filters from “links” and “copyedit” to just “links”. For wikis that don’t have unstructured link tasks, all those users get “add a link” and in that case we’ll exclude that wiki from the experiment.
We plan to continue to have a Control group that does not get access to the Growth features, which is a randomly selected 20% of new registrations.
- Group A: users get “add a link” as their only default task type.
- Group B: users get unstructured link task as their only default task type.
- Group C: control (no Growth features)
The experiment will run for a limited time, most likely between four to eight weeks. In practice the experiment will start with our four pilot wikis. After two weeks, we will analyze the leading indicators below to decide whether to extend the experiment to the rest of the Growth wikis.
Experiment Analysis and Findings
After completion of "Add a link" Experiment Analysis, we can conclude that the "add a link" structured task improves outcomes for newcomers over both a control group that did not have access to the Growth features as well as the group that had the unstructured "add links" tasks, particularly when it comes to constructive (non-reverted) edits. The most important points are:
- Newcomers who get the Add a Link structured task are more likely to be activated (i.e. make a constructive first article edit).
- They are also more likely to be retained (i.e. come back and make another constructive article edit on a different day).
- The feature also increases edit volume (i.e. the number of constructive edits made across the first couple weeks), while at the same time improving edit quality (i.e. the likelihood that the newcomer's edits are reverted).
Leading Indicators and Plan of Action
We are at this point fairly certain that Growth features are not detrimental to the wiki communities. That being said, we also want to be careful when experimenting with new features. Therefore, we define a set of leading indicators that we will keep track of during the early stages of the experiment. Each leading indicator comes with a plan of action in case the defined threshold is reached, so that the team knows what to do.
Indicator | Plan of Action |
---|---|
Revert rate | This suggests that the community finds the Add a Link edits to be unconstructive. If the revert rate for Add a Link is significantly higher than that of unstructured link tasks, we will analyze the reverts in order to understand what causes this increase, then adjust the task in order to reduce the likelihood of edits being reverted. |
User rejection rate | This can indicate that we are suggesting a lot of links that are not good matches. If the rejection rate is above 30%, we will QA the link recommendation algorithm and adjust thresholds or make changes to improve the quality of the recommendations. |
Task completion rate | This might indicate that there’s an issue with the editing workflow. If the proportion of users who start the Add a Link task and complete it is lower than 75%, we investigate where in the workflow users leave and deploy design changes to enable them to continue. |
Analysis and Findings
We collected data on usage of Add a Link from deployment on May 27, 2021 until June 14, 2021. This dataset excluded known test accounts, and does not contain data from users who block event logging (e.g. through their ad blocker).
This analysis categorizes users into one of two categories based on when they registered. Those who registered prior to feature deployment on May 27, 2021 are labelled "pre-deployment", and those who registered after deployment are labelled "post-deployment". We do this because users in the "post-deployment" group are randomly assigned (with 50% probability) into either getting Add a Link or the unstructured link task. Users in the "pre-deployment" group have the unstructured link task replaced by Add a Link. By splitting into these two categories, we're able to make meaningful comparisons between Add a Link and the unstructured link task, for example when it comes to revert rate.
Revert rate: We use edit tags to identify edits and reverts, and reverts have to be done within 48 hours of the edit. The latter is in line with common practices for reverts.
User registration | Task type | N edits | N reverts | Revert rate |
---|---|---|---|---|
Post-deployment | Add a Link | 290 | 28 | 9.7% |
Unstructured | 63 | 22 | 34.9% | |
Pre-deployment | Add a Link | 958 | 49 | 5.1% |
For the post-deployment group, a Chi-squared test of proportions finds the difference in revert rate to be statistically significant (). This means that the threshold described in the leading indicator table is not met.
Rejection rate: We define an "edit session" as reaching the edit summary or skip all dialogue, at which point we count the number of links that were accepted, rejected, or skipped. Users can reach this dialogue multiple times, because we think that choosing to go back and review links again is a reasonable choice.
User registration | N accepted | % | N rejected | % | N skipped | % | N total |
---|---|---|---|---|---|---|---|
Post-deployment | 597 | 72.4 | 125 | 15.2 | 103 | 12.5 | 825 |
Pre-deployment | 1,464 | 65.1 | 595 | 26.5 | 189 | 8.4 | 2,248 |
2,061 | 67.1 | 720 | 23.4 | 292 | 9.5 | 3,073 |
The threshold in the leading indicator table was a rejection rate of 30%, and this threshold has not been met.
Over-acceptance rate: This was not part of the original leading indicators, but we ended up checking for it as well in order to understand whether users were clicking "accept" on all the links and saving those edits. We reuse the concept of an "edit session" from the rejection rate analysis, and count the number of users who only have sessions where they accepted all links. In order to understand whether these users make many edits, we measure this for all users as well as for those with five or more edit sessions. In the table below, the "N total" column shows the total number of users with that number of edit sessions, and "N accepted all" the number of users who only have edit sessions where they accepted all suggested links.
User registration | N total | N accepted all | % | |
---|---|---|---|---|
Post-deployment | ≥1 edit | 96 | 31 | 32.3 |
≥5 edits | 6 | 0 | 0.0 | |
Pre-deployment | ≥1 edit | 64 | 10 | 15.6 |
≥5 edits | 19 | 1 | 5.3 |
We find that some users only have sessions where they accepted all links, but these users do not typically continue to make Add a Link edits. Instead, users who make additional edits start rejecting or skipping links as needed.
Task completion rate: We define "starting a task" as having an impression of "machine suggestions mode". In other words, the user is loading the editor with an Add a Link task. "Completing a task" is defined as clicking to save an edit, or confirming that all suggested links were skipped.
User registration | N Started a Task | N Completed 1+ Tasks | % |
---|---|---|---|
Post-deployment | 178 | 96 | 53.9 |
Pre-deployment | 101 | 64 | 63.4 |
279 | 160 | 57.3 |
The threshold defined in the leading indicator table is "lower than 75%", and this threshold has been met. In this case, we're planning to do follow-up analysis to understand more about the tasks, e.g. if they had a low number of suggested links, or if this happens on specific wikis or platforms.
Rejection Reasons
We've analyzed data on why users reject suggested links, focusing on newcomers to help us understand how they learn what constitutes good links in Wikipedia. In this analysis, we used rejections from January and February 2022, and restricted it to actions made within 7 or 28 days since registration. There was no significant difference in patterns between the two, and the data reported here uses the 28 day window. The data was split by wiki, platform (desktop or mobile) and bucketed by the number of Add a Link edits the user had made. We used a logarithmic bucketing scheme with 2 as the base, because that gives us a fair number of buckets early in a user's life while at the same time being easy to understand since the limits double each time.
Platform | Rejection reason | N | % |
---|---|---|---|
Desktop | Almost everyone knows what it is | 2,732 | 53.0% |
Linking to wrong article | 1,377 | 26.7% | |
Other | 688 | 13.0% | |
Text should include more or fewer words | 378 | 7.3% | |
Mobile | Almost everyone knows what it is | 1,835 | 53.3% |
Linking to wrong article | 791 | 23.0% | |
Other | 484 | 14.1% | |
Text should include more or fewer words | 271 | 7.9% | |
Undefined | 62 | 1.8% |
The distribution of these reasons is generally the same across all wikis, platforms, and number of Add a Link edits made. For some combinations of these features, we run into the issue of having few data points available (e.g. because some wikis lean strongly to usage of one platform) and that might result in a somewhat different distribution (e.g. just ones marked "Text should include more or fewer words"). In general, we have a lot of data for users with few edits as that's what most users are.
One thing we do appear to see is that for some wikis the usage of "Other" decreases as the number of Add a Link edits made increases. We interpret this to mean that "Other" might be a catchall/safe category for less experienced users, and that as they become more experienced and confident in labelling a link they'll use a different category.
Engineering
Link recommendation algorithm
See this page for an explanation of the link recommendation algorithm and for statistics around its accuracy. In short, we believe that users will experience an accuracy around 75%, meaning that 75% of the suggestions they get should be added. It is possible to tune this number, but the higher the accuracy is, the fewer candidate link we will be able to recommend. After the feature is deployed, we can look at revert rates to get a sense of how to tune that parameter.
For a detailed understanding of how the algorithm functions and is evaluated, see this research paper.
Link recommendation service backend
To follow along with engineering progress on the backend "add link" service, please see this page on Wikitech.
Articles selection
Articles are selected based on the topic(s) the use chooses. Then articles with a low ratio of links compared to the number of words are selected. A small amount of randomness was added to it so that not every article suggested look the same. The formula is documented on Phabricator.
Deployment
On May 27, 2021, we deployed the first iteration of this task to our four pilot wikis: Arabic, Czech, Vietnamese, and Bengali Wikipedias. It is available to half of new accounts, as described above. All accounts created before the deployment will also have the feature available. After two weeks, we will analyze our leading indicators to determine if any quick changes need to be made. After about four weeks, we will use data and community feedback to determine whether and how to deploy the feature to more wikis.
Since the initial deployment to our pilot wikis, the Growth team has gathered extensive community feedback and data about the usage and value of Add a Link. We then used those learnings to make improvements to the feature so that newcomers would have a better experience and experienced editors would see higher quality edits. We have completed the improvements, and communities are now using iteration 2 of Add a Link.
The deployment to all Wikipedias was completed at the end of 2023, with only few wikis missing: German and English as they require specific community engagement, and very small Wikipedias where there is no critical mass to have enough links.